Predictive maintenance (PdM) always seemed like the perfect use case for the Internet of Things (IoT), more specifically for Industrial IoT (IIoT) and environments where uptime of specific assets is critical and breakdowns can have important consequences for several reasons.
No wonder that predictive maintenance is one of the most often mentioned use cases in Industry 4.0. PdM isn’t just about smart manufacturing, though. Industries/segments with predictive maintenance as a use case further include transportation, oil and gas, process industries overall, and ample segments with critical power environments. Simply put: where the scenarios and use cases are where PdM makes the most – business value – sense.
The cornerstone of success of predictive maintenance is the prediction accuracy and connection of the predictive model outputs with the enterprise maintenance processes (Jan Burian, IDC Manufacturing Insights)
Many of the main technologies which are often cited in the context of Industry 4.0 (can) play a role in predictive maintenance and its evolutions: big data, AI and ML (artificial intelligence and machine learning), IoT, cloud computing, data analytics and, increasingly, edge computing and digital twins.
IIoT, in combination with predictive analytics and ML, however, are the main drivers of the more mature stage of predictive maintenance.
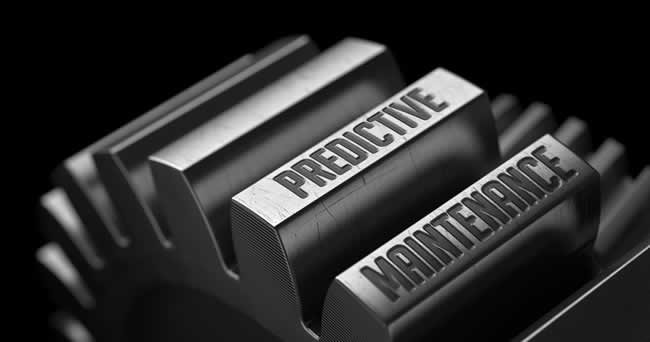
Actionable, often real-time, data on pre-defined factors are gathered from smart sensors and predictive analytics algorithms are applied to predict when something might occur – and thus proactive maintenance, called predictive in this case, is needed.
The idea behind predictive maintenance is simple and attractive enough and is similar to other forms of proactive maintenance with some additional benefits (and disadvantages): unexpected failure of equipment with all related consequences can be avoided, and maintenance can occur before something happens, instead of after the facts (reactive and run-to-failure maintenance), when such is deemed useful and possible. So, predictive maintenance is one of the maintenance methods enabling to do this kind of proactive maintenance and takes most time and skills to implement. In other words: it’s important to use it where it makes true sense. Predictive maintenance certainly isn’t the holy grail of maintenance, it has its limits too.
Predictive maintenance in the scope of maintenance 4.0
The usage of predictive maintenance is less common-place than providers of solutions would like, and customers, except for specific verticals and segments, so far have been slower to adopt it than was expected in the earlier days of the Internet of Things. However, things are starting to change again.
In the scope of this article, we define predictive maintenance as what is also known as Maintenance 4.0, or PdM 4.0, in correlation with the mentioned technologies and related systems (e.g., CMMS or Computerized Maintenance Management System).
Due to advances in AI and the increase in data points, sophisticated analytics are becoming more and more important and make up a larger share of the overall predictive maintenance budget (IoT Analytics)
The reason: predictive maintenance as such isn’t new and in the traditional sense not even necessarily related to these technologies at all since simple – human visual and instrument – inspections also enable predictive maintenance. With IIoT, machine learning and so forth what is now often called predictive maintenance is in fact a more advanced stage of PdM, with more data sources. Others will use condition-based monitoring and predictive maintenance interchangeably.
Yet, along with visual inspections and instrument inspections, real-time condition monitoring is seen as an evolution in PdM whereby the more ‘modern’ and mature state of predictive maintenance as said has, among others, been called PdM 4.0 (it is not the only model), with the 4.0 obviously referring to Industry 4.0 and its smart factory, and with PdM 4.0 as this fourth stage of maturity in the evolution of predictive maintenance if you will.
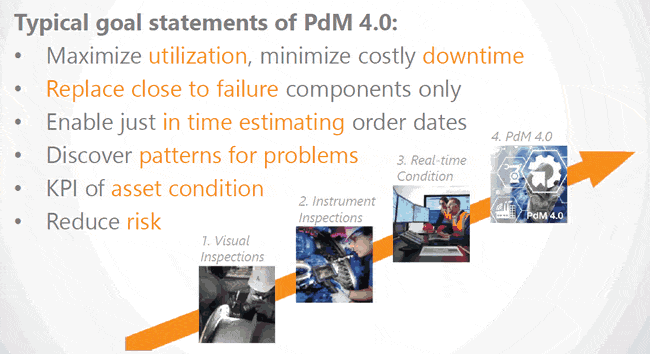
The downsides of reactive maintenance (a.k.a. breakdown maintenance) whereby equipment/assets get repaired after having broken down are clear enough. This doesn’t mean that reactive maintenance is bad of course.
Well, on the contrary, reactive maintenance in many cases is part of a strategic choice and the type of maintenance depends on many factors such as the consequences of equipment failure and whether it’s commercially or otherwise interesting to invest in more proactive maintenance methods or not (and if so, in which one). It’s always a mix and sometimes predictive maintenance won’t even be possible, let alone a good option.
Yet, with assets that are critical and need to be available at all times for one or the other reason (ample examples where equipment failure can result in astronomic costs, huge reputation damage or even worse), alternative options are better, even if they come with more planning and up-front investments. It all depends on the importance of the asset from a broader perspective.
Condition monitoring and predictive maintenance of the complex production assets are still far from plug and play solutions
Predictive maintenance is also just one of those means to act before something breaks down (proactive maintenance), rather than after the facts. Another form of proactive maintenance is preventive maintenance that also focuses on decreasing the chance that something goes wrong.
Sometimes such maintenance approaches will be combined with redundancy approaches whereby organizations look at ways to continue operations, even if something does break down. This can, for example, be the case in environments where the stakes are really high. Example: as Aimen Abd-el-azim explains in an interview on substation automation, to make sure the entire electrical network is working within the right environment and optimal conditions, in critical infrastructures, the main hardware is often duplicated with different algorithms (from other vendors) to ensure one will operate if the second fails or is temporarily out of service.
PdM 4.0 – the next stage after condition monitoring in predictive maintenance
As said, predictive maintenance is often called condition monitoring but real-time condition monitoring is seen as an earlier maturity stage in the bigger predictive maintenance monitoring picture of PdM 4.0.
We won’t elaborate too much on that for now since both condition-based monitoring and predictive monitoring as it’s understood today are far from omnipresent but if you want to know more check out this blog post by Jan Burian, Research Director, IDC Manufacturing Insights on the changing role of enterprise maintenance in manufacturing.
Condition monitoring and predictive maintenance compared in a nutshell:
Condition monitoring looks at pre-defined parameters (e.g., with vibration analysis and diagnostics, oil analysis, motor condition monitoring and motor current signature analysis, thermal analysis, or whatever type of technique that’s relevant for the monitored equipment) and compares the parameters with predefined thresholds whereby through visualization the condition of the machine can be checked.
Predictive maintenance uses ML engines with these parameters of the monitored equipment being used as a basis, but the actual difference concerning what the machine learning and predictions say what is likely to occur within a specific time frame and with a specific probability. Typically, this also means that predictive maintenance uses more data sources and sets than the sensor data from condition monitoring and digitally recorded data from the previous stage, instrument inspection.
The difference might seem small, but it’s not. Predictive maintenance uses monitoring data, but it uses more and, most importantly, does more with the data. It, indeed, predicts.
The presentation at the bottom of this blog goes a bit more in detail and makes the differences more tangible with additional information on what to consider when implementing predictive maintenance.
The predictive maintenance market and some words of advice
IoT Analytics expects the market for predictive maintenance solutions to reach $23.5B by 2024.
The graphic below shows that it is further estimated that in 2024, adopters of PdM will save $188B in costs on top of other benefits such as improved regulatory compliance and enhanced safety. You can also see some of the challenges and shifts concerning the adoption of predictive maintenance.
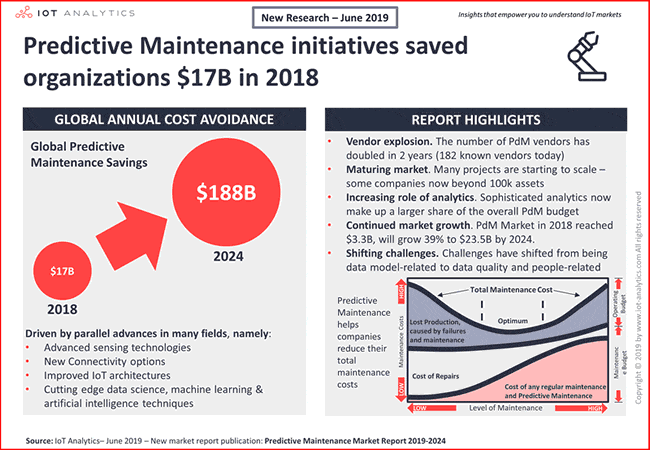
Obviously, there is much more research out there on the market. Stay tuned for more details, also concerning the industries that use PdM most with additional strategic advice regarding the usage of predictive maintenance and in the meantime check out the promised presentation below that is largely based on work from PwC.
Do take into account that several challenges had a negative impact on the adoption of PdM, however, which is food for a next contribution. For now, let’s conclude with some words of advice from IDC’s Jan Burian for the decision makers among you: “Condition monitoring and predictive maintenance of the complex production assets are still far from plug and play solutions. Detailed preparation on customization and final fine-tuning are crucial success factors. Deploy the advanced condition monitoring first and then look to where the predictive models could be deployed. Think about building digital twins of the assets and process and combine data from the asset aggregates, production process, and production environment”. More in his article.
Choosing the right maintenance strategy starts with understanding the various types, and there are quite a few indeed. On top of preventive and predictive maintenance, there is reactive (run-to-failure), risk- and condition-based, reliability-centered, total productive maintenance and even more.
Data and preparing for predictive maintenance
The precise mix of maintenance strategies depends on the assets and their place and importance in various scenarios. It’s clear that with predictive maintenance data is essential from all perspectives: the gathering and analysis of data, the various data sources and ability to leverage them and integrate with existing systems, the available skills with regards to data, the needed data systems and the quality of data.
If you’re not using predictive maintenance in the sense of PdM 4.0 yet but consider doing so in the future when the business case is clearer, already start storing the data which would be relevant once you effectively go for PdM.
A lack of data skills and issues concerning data quality are important challenges for the deployment of predictive maintenance
Obviously it’s not just about gathering. One of the big challenges in predictive maintenance, given the various data sources and the involvement of, for instance, the OEM of the machine, is the combination of all the data sets to be leveraged for predictive maintenance. Often data gets gathered but then remains in a data lake without any action being taken. In fact, one of the main reasons why organizations aren’t ready for PdM 4.0 is that they don’t use the data they already have, still relying on Excel, for example, and thus see no use in gathering even more data.
Gathering data is one thing, integration, and especially being able to derive the needed insights and value another. This is also the reason why predictive maintenance hasn’t taken been as widely adopted as expected so far as research indicates.
In order to make predictive maintenance succeed, the organization – and departments (IT, data scientists, business, operators,…) and partners involved – need to dispose of the necessary skills on the level of big data technologies, business intelligence, data warehousing etc. Moreover, and this is crucial, there must be an unconditional focus on data quality.
Read more about other elements that are key to tun a successful predictive maintenance program via the button below: from top management support to key performance indicators.